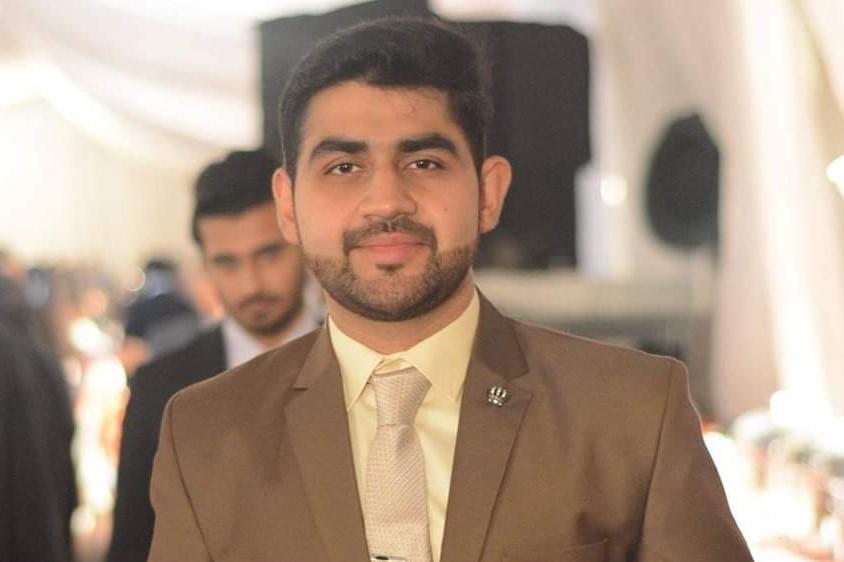
Modelling Battery Degradation and Estimating Battery Life in Swappable EV Technology using Machine Learning
Electric vehicle technologies employ lithium-ion batteries as a vehicle power source and the electrical energy storage device. Lithium-ion batteries are presently pervasive in EV swappable technology and they must be meticulously monitored and manipulated, where effectively estimating their state of health is a key enabler. However, one main limitation of these technologies resides in the battery ageing. EVs employing lithium-ion battery are facing difficulties in terms of ageing induced degradation, and predicting accurate health and remaining useful life states due to various internal and external factors. Battery degradation takes place in every condition, but in different proportions, as usage and external conditions interact to provoke degradations. The degradation challenges are resolved by the use of quantitative diagnosis based on the insights which are provided for developing online battery ageing diagnosis and battery health management. To ensure batteries are reliable, efficient, and capable of delivering power and energy when required, an accurate determination of battery performance, health, and life prediction are necessary. Estimation strategies play an important role in battery health management and various estimation strategies have been developed to monitor battery health status and performance, along with the evolution of prognostics modelling methods. The goal of these strategies is to render feasible and cost-effective solutions for dealing with battery life issues under dynamic operating conditions. Recent advancements in data analytics and related statistical tools raised interest in data-driven battery health estimation. The feasibility and cost-effectiveness of data-driven techniques to deal with the challenges of real-time battery health management have boosted progress in battery health estimation and prediction in real-world applications. Among the data-driven techniques, machine learning (ML) is becoming more popular for estimating the state of health (SOH) due to greater availability of battery data and improved computing power capabilities. In our study, different ML models are deployed depending on the data quality, inputs and outputs, test conditions, battery types, and stated accuracy to have a bigger picture view of the ML-based SOH estimation. In our study, a conceptual framework for battery degradation modelling is proposed using ML techniques that can be easily used in EV studies. We also proposed research and development perspectives to conduct further studies on the swappable Battery based EVs and the battery operation and health monitoring, including the approach selection, health management, performance evaluation, application economics, as well as environmental issues.
Zoom Link: https://zoom.us/j/99977446436?pwd=UndYSWxtRkI0aTgrRGh3bkZkVVgwUT09
Meeting ID: 999 7744 6436
Passcode: 686401
Publications
Journals:
H. Rauf, M.S. Gull, and N. Arshad "Complementing hydroelectric power with floating solar PV for daytime peak electricity demand," Renewable Energy, Volume 162, Pages 1227-1242, 2020. https://www.sciencedirect.com/science/article/pii/S0960148120312544
H. Rauf, M.S. Gull, and N. Arshad" Integrating floating solar PV with hydroelectric power plant: analysis of Ghazi Barotha reservoir in Pakistan, Energy Procedia, Volume 158, Pages 816-821, February 2019, https://www.sciencedirect.com/science/article/pii/S1876610219302243
H. Mansoor, H. Rauf, M. Mubashir, M. Khalid, and N. Arshad " Past Vector Similarity for Short Term Electrical Load Forecasting at the individual Household Level," IEEE Access (Accepted)
H. Rauf, H. A. Khan, and N. Arshad, " Optimized Power System Planning for Base Transceiver Station (BTS) based on Minimized Power Consumption and Cost " in 2019 8th International Conference on Renewable Energy Research and Applications (ICRERA): IEEE, pp. 773-779. https://ieeexplore.ieee.org/abstract/document/8997043