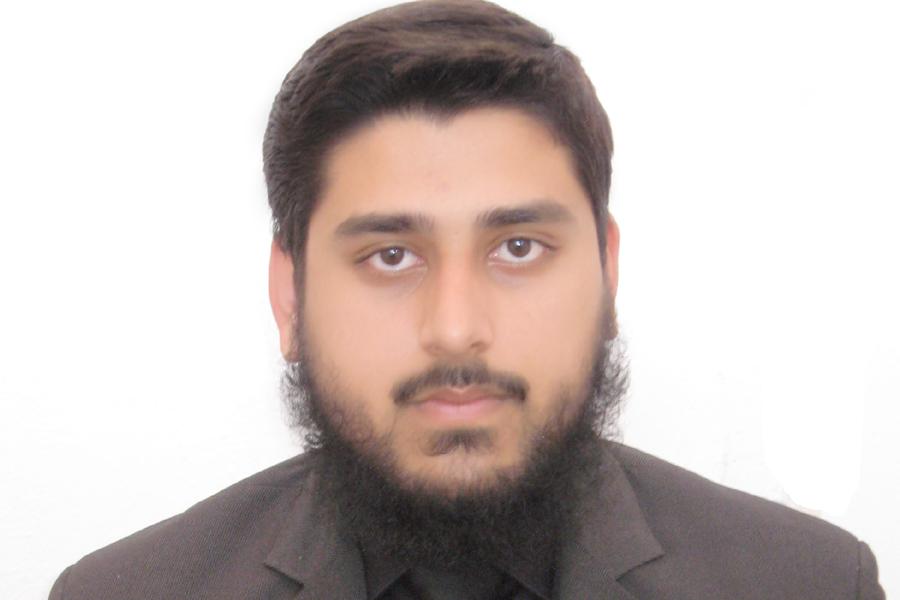
Data-Driven Approaches for Context-Aware Sports Performance Assessment and Web Energy Optimization
Abstract:
Data analytics plays a critical role in enabling evidence-based decision-making, uncovering hidden patterns, and providing a competitive advantage to organizations in various fields, such as sports, business, finance, healthcare, marketing, and more. Our work makes substantial contributions to the advancement of data analytics and modeling techniques across a wide range of domains, spanning from the evaluation of sports performance to enhancing the energy efficiency of web applications. We propose context-aware data-driven performance assessment and energy optimizations for the sports and web domain.
Cricket is the second most popular sport after soccer in terms of viewership. However, the assessment of individual player performance, a fundamental task in team sports, is currently primarily based on aggregate performance statistics, including average runs and wickets taken. We propose Context-Aware Metric of player Performance, CAMP, to quantify individual players’ contributions toward a cricket match outcome. CAMP employs data mining methods and enables efficient, unbiased, and data-driven decision-making for selection and drafting, coaching and training, team line-ups, and strategy development. CAMP incorporates the exact context of performance, such as opponents’ strengths and specific circumstances of games, such as pressure situations. We empirically evaluate CAMP on data of limited-over cricket matches between 2001 and 2019. In every match, a committee of experts declares one player as the best player, called Man of the Match (MOM). The top two rated players by CAMP match with MOM in 83% of the 961 games. Thus, the CAMP rating of the best player closely matches that of the domain experts. By this measure, CAMP significantly outperforms the current best-known players’ contribution measure based on the Duckworth-Lewis-Stern (DLS) method.
Battery-constrained mobile devices generate significant web traffic globally, underscoring the critical need for energy-efficient web applications. While load-time performance metrics of web applications can be used to quantify their energy consumption, performance is not always correlated with power requirements due to device and communication network variations. Addressing this issue, we propose a novel approach to model the load-time power consumption of web apps by leveraging their static design features. Unlike performance matrices, The static features are invariant to the underlying device, platform, and network. The proposed model provides valuable high-level insights into the expected power consumption of web apps, enabling developers to make informed feature adjustments during the design phase to enhance energy efficiency. We empirically evaluate the effectiveness of our approach using the design features and load-time power consumption data of 1000 benchmark websites. Our predictive model achieves a notable 9.7% gain in accuracy and a 5.7% gain in AUC-ROC compared to the performance-based predictive model. Furthermore, we curated websites’ features and power consumption data using software tools and made the cleaned and pre-processed data publicly available, facilitating further research in this domain. Our work contributes to developing more sustainable web applications and provides developers with actionable insights to optimize power consumption, ultimately supporting the goal of reducing the environmental impact of the digital ecosystem.
Both sub-problems, spanning cricket and web applications, pertain to performance evaluation in distinct domains. Existing methods reliant on aggregate statistics or performance metrics exhibit limitations in capturing genuine contributions or energy efficiency. This thesis endeavors to advance performance assessment in cricket by accurately quantifying individual player contributions in web applications by precisely quantifying energy consumption. Through innovative approaches tailored to each domain's unique contexts and features, this research enriches the comprehension and enhancement of performance assessment in cricket and web application development.
Final Defense Committee (FDC):
Dr. Mian Muhammad Awais (Thesis Committee Member)
Professor, Department of Computer Science, Lahore University of Management Sciences (LUMS), Pakistan.
Dr. Basit Shafiq (Thesis Committee Member)
Associate Professor, Department of Computer Science, Lahore University of Management Sciences (LUMS), Pakistan.
Dr. Imdad Ullah Khan (PhD Supervisor)
Associate Professor, Department of Computer Science, Lahore University of Management Sciences (LUMS), Pakistan.
Dr. Imran Anwar (Internal Examiner)
Associate Professor, Department of Mathematics, Lahore University of Management Sciences (LUMS), Pakistan.
Dr. Mohsen Ali (External Examiner)
Associate Professor, Department of Computer Science, Information Technology University (ITU), Lahore, Pakistan.
Dr. Safiullah Faizullah (External Examiner)
Professor, Department of Computer Science, Islamic University of Madinah, KSA.
Publications:
Journal Publications:
[1] Ayub, M. S., Ullah, N., Ali, S., Khan, I. U., Awais, M. M., Khan, M. A., & Faizullah, S. (2023). CAMP: A context-aware cricket players performance metric. Journal of the Operational Research Society, 1-17. (https://www.tandfonline.com/doi/full/10.1080/01605682.2023.2237530)
[2] Ayub, M. S., Mustafa, N., Ali, S., Ahmad, J., Khan, M. A., Faizullah, S., & Khan, I. U. Modeling Power Consumption of Web Apps using Static Design Features. (Under Review)
Conference Publications:
[3] Ayub, M. S., & Siddiqui, J. H. (2018, May). Efficiently finding minimal failing input in MapReduce programs. In Proceedings of the 40th International Conference on Software Engineering: Companion Proceeedings (pp. 177-178). (https://ieeexplore.ieee.org/document/8449488)
[4] Ayub, M. S., Rehman, W. U., & Siddiqui, J. H. (2017, October). Experience report: Verifying mpi java programs using software model checking. In 2017 IEEE 28th International Symposium on Software Reliability Engineering (ISSRE) (pp. 294-304). IEEE. (https://doi.org/10.1109/ISSRE.2017.15)
[5] Rehman, W. U., Ayub, M. S., & Siddiqui, J. H. (2016, February). Verification of MPI Java programs using software model checking. In Proceedings of the 21st ACM SIGPLAN Symposium on Principles and Practice of Parallel Programming (pp. 1-2). (https://doi.org/10.1145/2851141.2851192)
Other Publications during PhD:
Journal Publications:
[6] Alam, S., Zhao, X., Niazi, I. K., Ayub, M. S., & Khan, M. A. (2023). A comparative analysis of global optimization algorithms for surface electromyographic signal onset detection. Decision Analytics Journal, 100294. (https://www.sciencedirect.com/science/article/pii/S2772662223001340)
[7] Faizullah, S., Ayub, M. S., Hussain, S., & Khan, M. A. (2023). A Survey of OCR in Arabic Language: Applications, Techniques, and Challenges. Applied Sciences, 13(7), 4584. (https://doi.org/10.3390/app13074584)
Conference Publications:
[8] Sardar, U., Ali, S., Ayub, M. S., Shoaib, M., Bashir, K., Khan, I. U., & Patterson, M. (2023). Sequence-Based Nanobody-Antigen Binding Prediction. International Symposium on Bioinformatics Research and Applications
[9] Alam, S., Pande, R., & Ayub, M. S., Khan M.A. (2023) Towards Developing an Automated Chatbot for Predicting Legal Case Outcomes: A Deep Learning Approach. Asian Conference on Intelligent Information and Database Systems
International Presentation and Demonstrations:
Poster Presentation with Demonstration in 40th International Conference on Software Engineering (ICSE), May 27 - 3 June 2018, Gothenburg, Sweden
Research Paper Presentation in 28th International Symposium on Software Reliability Engineering (ISSRE) October 23-26, 2017 Toulouse, France
Google Scholar: https://scholar.google.com.pk/citations?user=5hSXz_AAAAAJ&hl=en (For other under-review papers)