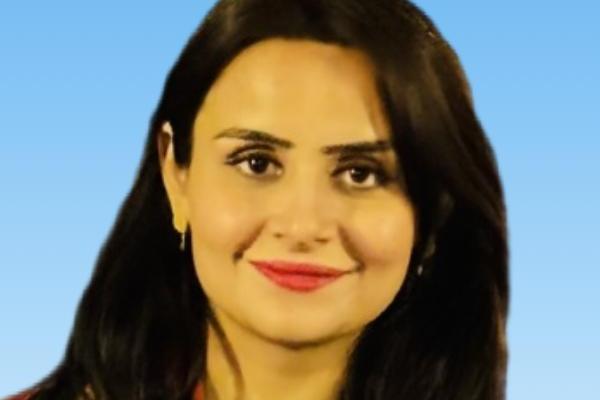
DeepIS-IPS: Optimizing Wi-Fi Indoor Positioning System with Deep Learning and Intelligent Surfaces
Abstract:
Indoor positioning remains challenging due to the limitation of Global Navigation Satellite Systems (GNSS) in indoor environments. Wi-Fi based Indoor Positioning Systems (IPS) presents a practical alternative that leverages the widespread availability of Wi-Fi indoors. However, multipath effects, interference, and minimal control over wireless signals often limit their accuracy. Achieving high accuracy in these systems typically requires Wi-Fi fingerprinting, which involves creating a comprehensive database of Wi-Fi signals and matching real-time data to estimate locations. IPS systems rely on increasing the number of Access Points (APs) to enhance dataset dimensionality, which is costly and challenging to scale. Additionally, acquiring and maintaining this fingerprinting database is labor-intensive, often requiring extensive RF measurements, crowdsourced data, or complex RF propagation models.
To address these limitations, this thesis introduces an advanced IPS framework that integrates Deep Learning (DL) models and Intelligent Surfaces (ISs), offering a scalable and adaptive solution that enhances control over the wireless environment. The research explores two systems: IPS without IS (NIS-IPS) and IPS with IS (IS-IPS). NIS-IPS employs Convolutional Neural Networks (CNNs) and an “RSSI Noise” model to capture and manage signal fluctuations accurately, achieving high precision with minimal recalibration. Virtual trajectories further adapt the system to environmental changes, reducing dependency on labor-intensive data collection. Experimental results validate that NIS-IPS significantly improves localization accuracy within existing infrastructure.
The IS-IPS approaches Reconfigurable Intelligent Surfaces (RIS) to dynamically reshape the Electromagnetic (EM) environment, enhancing control over wireless signals and overcoming the limitations of traditional IPS. By adjusting the phases of RIS unit cells, IS-IPS can achieve enhanced system dimensionality with a single AP and multiple RIS configurations, eliminating the need for additional APs. This RIS-assisted IPS employs a hybrid approach, combining analytical models with full-wave simulations to optimize real-time signal paths. Experimental validation demonstrates that RIS configurations enable precise localization without extensive fingerprinting databases,
significantly improving accuracy and scalability. Next, Stacked Intelligent Metasurfaces (SIM), inspired by the concepts of RIS and Deep Neural Networks (DNNs), employ layered EM control for enhanced localization precision. SIM-IPS divides indoor spaces into zones, utilizing optimized algorithms for zone identification configurations to achieve coarse localization, while fine localization is achieved through precise configurations. Simulations confirm substantial accuracy improvements through optimizing layers and zones, establishing SIM-IPS as a robust solution for high-precision indoor localization.
This thesis introduces an innovative IPS framework that addresses the core challenges of indoor positioning by integrating DL models with ISs. Both NIS-IPS and IS-IPS demonstrate substantial improvements in localization accuracy, minimize the need for frequent calibration, and offer enhanced scalability. These advancements effectively reduce the labor-intensive demands of traditional fingerprinting methods, meeting the growing need for reliable and efficient indoor positioning solutions.
Final Thesis Defense Committee:
1- Dr. Hassan-Mohy-Ud-Din (Associate Professor, Thesis Committee Member), Department of Electrical Engineering, Lahore University of Management Sciences (LUMS), Pakistan
2- Dr. Ijaz Haider Naqvi (Associate Professor, Thesis Committee Member), Department of Electrical Engineering, Lahore University of Management Sciences (LUMS), Pakistan
3- Dr. Basit Shafique (Associate Professor, Thesis Committee Member), Department of Computer Science, Lahore University of Management Sciences (LUMS), Pakistan
4- Dr. Muhammad Qasim Mehmood (Associate Professor, External Examiner), Department of Electrical Engineering, Information Technology University (ITU), Lahore, Pakistan
5- Dr. Naveed Ul Hassan (Associate Professor, PhD Supervisor), Department of Electrical Engineering, Lahore University of Management Sciences (LUMS), Pakistan
List of Publications:
Journal Papers:
1. Aisha Javed, Naveed Ul Hassan, Ammar Rafique, Muhammad Zubair, Marco Di Renzo, and Chau Yuen, "Fingerprinting Database Development Methods for Reconfigurable Intelligent Surface Assisted Indoor Positioning System," in IEEE Access, vol. 12, pp. 85244–85258, 2024.
2. Aisha Javed, Naveed Ul Hassan, Marco Di Renzo, and Chau Yuen, "SIM-IPS: Zone-Based Optimization for Accurate Indoor Positioning Using Multilayer Intelligent Metasurfaces," manuscript under preparation for submission to IEEE Internet of Things Journal.
Conference Papers:
3. Aisha Javed, Naveed Ul Hassan, and Chau Yuen, "Accurate and Stable Wi-Fi based Indoor Localization and Classification Using Convolutional Neural Network," 2021 IEEE VTS 17th Asia Pacific Wireless Communications Symposium (APWCS), IEEE, 2021, pp. 1–5.
4. Aisha Javed and Naveed Ul Hassan, "Low-Effort Deep Learning Method Trained through Virtual Trajectories for Indoor Tracking," 2021 IEEE 32nd Annual International Symposium on Personal, Indoor and Mobile Radio Communications (PIMRC), IEEE, 2021, pp. 1546–1551.
5. Aisha Javed, Ammar Rafique, Muhammad Zubair, and Naveed Ul Hassan, "Revisiting End-to-End Communication Model for Realistic Reconfigurable Intelligent Surfaces," 2022 IEEE International Symposium on Antennas and Propagation and USNC-URSI Radio Science Meeting (AP-S/URSI), IEEE, 2022, pp. 1868–1869.