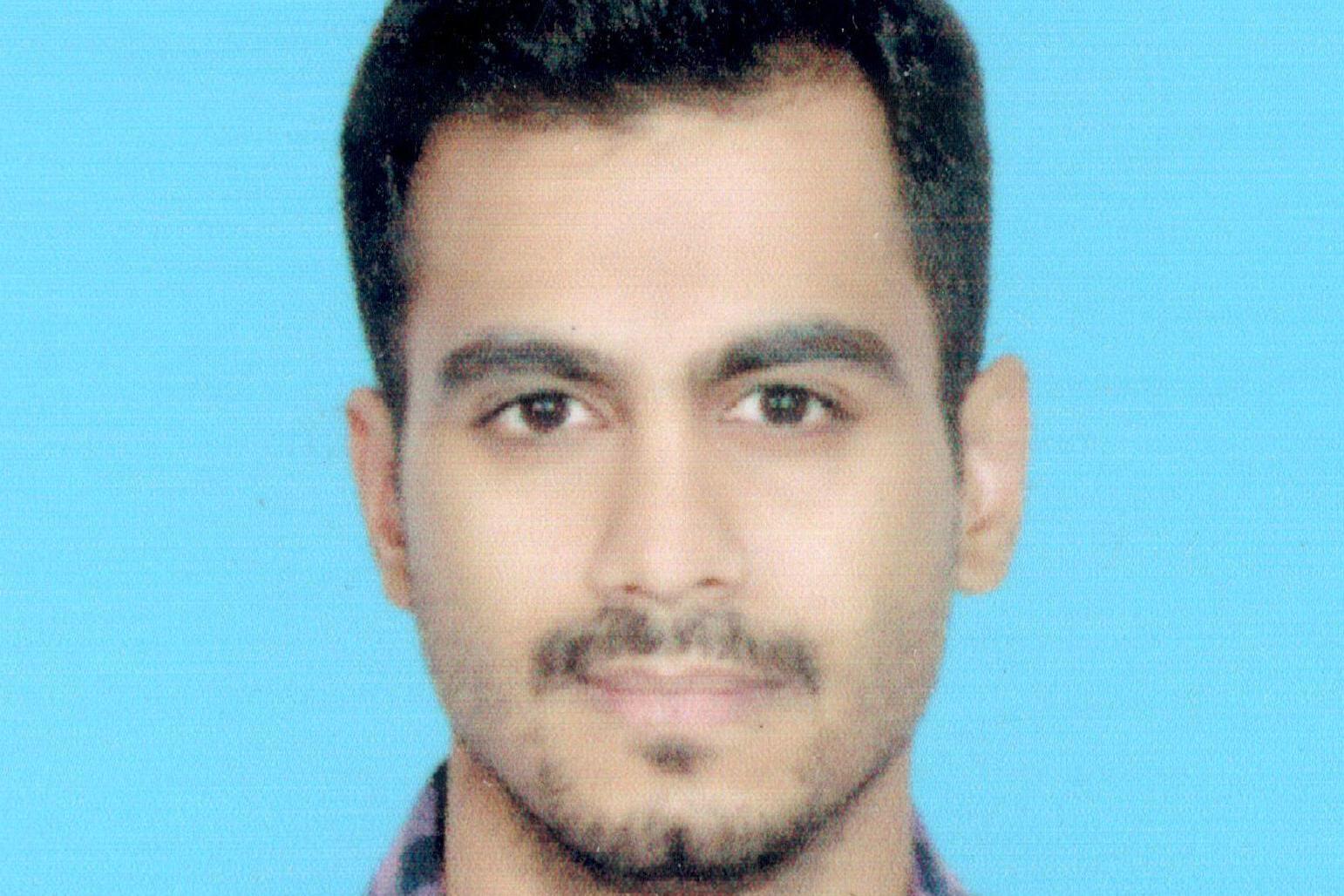
Robust Filtering – Novel Statistical Learning and Inference Algorithms with Applications
Abstract
Filtering or state estimation of dynamical systems is a crucial inference task for providing new decision-making and system automation information in different applications. Examples include energy management systems, smart grids, robotics, healthcare, intelligent transportation systems, etc. The standard filtering setup assumes that the system dynamics are adequately captured by state space models, where the latent states evolve with first-order dynamics. Though not directly observable, the states manifest as a set of noisy external outputs measured by sensors. Considering the requirement of an online recursive solution in addition to the possibilities of underlying functional nonlinearities and dependency on noisy observations for inference the filtering problem is not trivial.
The complexity of filtering is compounded when the measurements get corrupted with different abnormalities or gross errors having unknown or partially known statistics. This violates the assumption of standard filtering approaches where the nominal noise statistics are assumed to be known apriori. Gross errors encompass disturbances apart from the nominal noise, including outliers and biases/drifts. Generally modelled with zero-mean statistics, outliers are sudden sporadic surges in the measurements. Their appearance can be attributed to factors like sensor degradation, communication failures, environmental influences, front-end processing limitations, etc. On the other hand, biases disturb the data with non-zero mean noise statistics. Multiple factors in various applications lead to the appearance of biased observations. Examples include miscalibrations or malfunctioning of sensors and other configuration aberrations like sensor location, alignment, clock errors, etc.
Mitigating the effect of measurement abnormalities on state estimation calls for robust filtering methods, which form the basis of this dissertation. Our primary emphasis remains on devising and investigating robust nonlinear filters considering the occurrence of outliers and biases in the data. In some cases, peripheral instances of missing observations and drifts are also evaluated. We also extend one of our proposed robust filters to a general estimation setup in the presence of outliers. Also a smoothing extension for a designed method is also presented. To make our devised methods implementable on digital computers, we restrict our focus to discrete-time state space models. By proposing suitable models, we develop and study different state estimation approaches. We resort to tools from statistical (mostly Bayesian) inference theory, including the deterministic and stochastic methods for distributional approximations. To gauge the theoretical estimation limitations, we evaluate the Bayesian Cramer Rao Bounds for some of our models. For practical performance evaluation, we choose a variety of appropriate simulations and experimental setups to gauge the gains compared to the state-of-the-art approaches mostly in terms of estimation accuracy and computational strains.
Final Thesis Defense Committee:
1- Dr. Imdad Ullah Khan (Associate Professor, Thesis Committee Member), Department of Computer Science, Lahore University of Management Sciences (LUMS), Pakistan
2- Dr. Hassan Jaleel (Assistant Professor, Thesis Committee Member), Department of Electrical Engineering, Lahore University of Management Sciences (LUMS), Pakistan
3- Dr. Ijaz Naqvi (Associate Professor, Thesis Committee Member), Department of Electrical Engineering, Lahore University of Management Sciences (LUMS), Pakistan
4- Dr. Khurram Ali (Associate Professor, External Examiner), Department of Electrical Engineering, Comsats, Lahore, Pakistan
5- Dr. Muhammad Tahir (Associate Professor, PhD Supervisor), Department of Electrical Engineering, Lahore University of Management Sciences (LUMS), Pakistan
6- Dr. Momin Uppal (Associate Professor, PhD Co-Supervisor), Department of Electrical Engineering, Lahore University of Management Sciences (LUMS), Pakistan
Publications
1- Chughtai, A. H., Akram, U., Tahir, M., & Uppal, M. (2020). Dynamic state estimation in the presence of sensor outliers using MAP-based EKF. IEEE Sensors Letters, 4(4), 1–4. https://ieeexplore.ieee.org/document/9050910
2- Chughtai, A. H., Tahir, M., & Uppal, M. (2020). A robust Bayesian approach for online filtering in the presence of contaminated observations. IEEE Transactions on Instrumentation and Measurement, 70, 1–15. https://ieeexplore.ieee.org/document/9239326
3- Chughtai, A. H., Tahir, M., & Uppal, M. (2022). Outlier-robust filtering for nonlinear systems with selective observations rejection. IEEE Sensors Journal, 22(7), 6887-6897. https://ieeexplore.ieee.org/document/9716131
4- Chughtai, A. H., Majal, A., Tahir, M., & Uppal, M. (2022). Variational-based nonlinear Bayesian filtering with biased observations. IEEE Transactions on Signal Processing. https://ieeexplore.ieee.org/document/9931968
5- Chughtai, A. H., Tahir, M., & Uppal, M. (2024). Bayesian heuristics for robust spatial perception IEEE Transactions on Instrumentation and Measurement. https://ieeexplore.ieee.org/document/10430181
6- Chughtai, A. H., Tahir, M., & Uppal, M. (2024). EMORF/S: EM-Based Outlier-Robust Filtering and Smoothing With Correlated Measurement Noise (Under Review). https://arxiv.org/abs/2307.02163