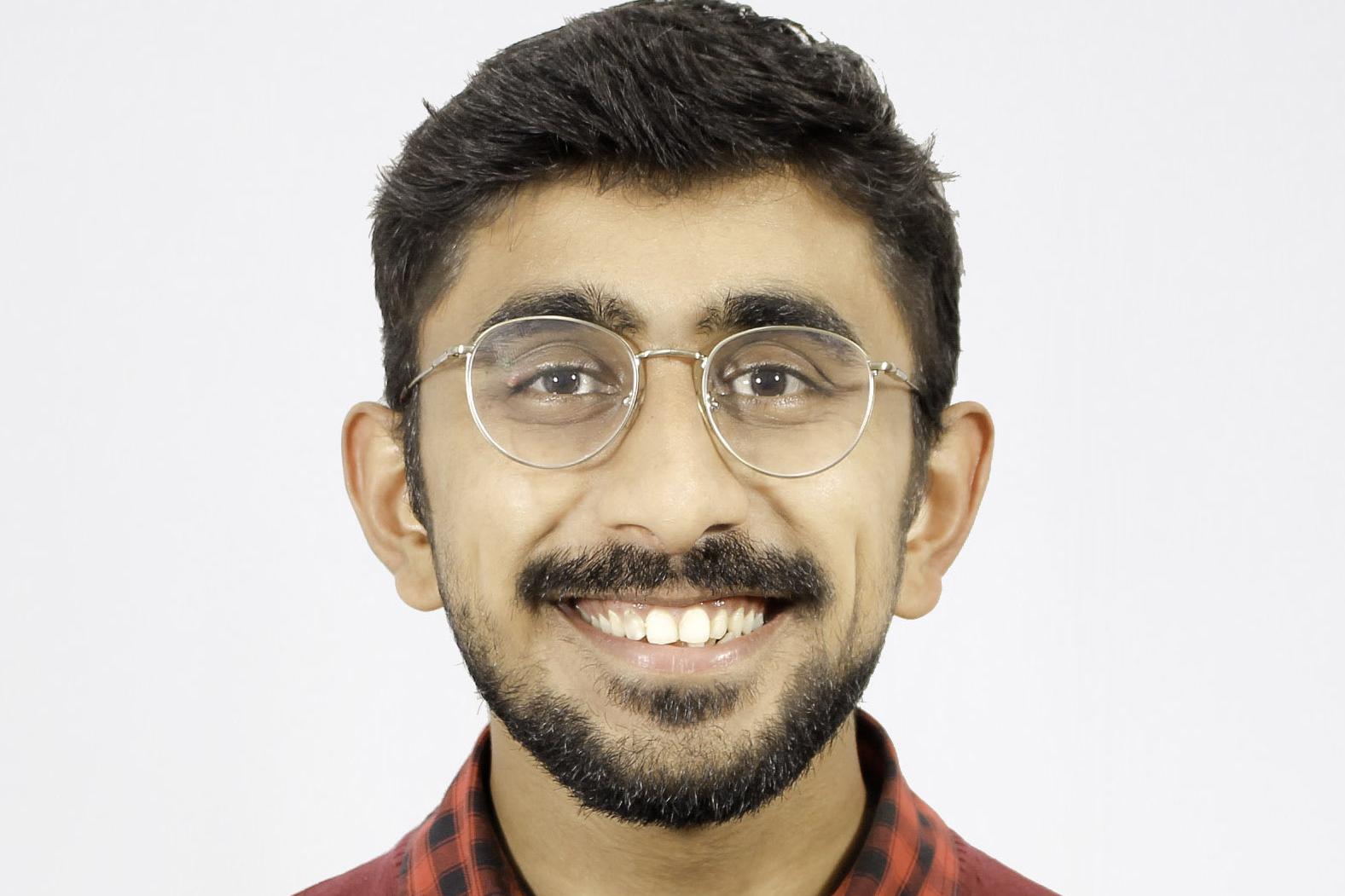
Event date:
Mar
8
2021
2:00 pm
Deep Learning Based 3D Reconstruction Using the Mobile LiDar Sensors
Supervisor
Dr. Murtaza Taj
Student
Muhammad Numan Minhas
Venue
Zoom Meetings (Online)
Abstract
The problem of 3D reconstruction has been a focus of the computer vision industry for the last few years because of the increased availability of different sensors. But the solutions explored by different researchers have been very diverse and there isn’t a straightforward way that most solutions use for problem formulation and benchmarking. There has been some research on the scanning side which mostly focused on using handheld sensors like Kinect. There are some datasets for Kinect based scanning, but these datasets mostly don’t have a ground truth because they are scanning a real-world object. On the other hand, most deep learning research on this problem is focused on using synthetic rendering data generated using ShapeNet models as ground truth. The major drawback of these techniques is that most affordable sensors have noisy scanning data while synthetic data is noise free. So, the data these models are trained on is fundamentally biased against noise in the input data. Furthermore, besides the general noise because of sensor artifacts, different types of sensor technologies have very specific types of noise, for example LiDar sensors have missing and noisy depth values around the borders of objects, they also have more noise around more shiny surfaces or around translucent surfaces. Similarly, the more distant a surface is, the more noise in its depth value there is. All this indicates that the deep learning models trained on synthetic data can most probably not be directly used for reconstructing models from scanning data. In this project, we will try to reconstruct a 3D mesh using data collected from a mobile phone scanner with LiDar sensor and see how their results compare on a 3D reconstruction task as compared to the results of a synthetic dataset.
Zoom Link: https://zoom.us/j/97696454919?pwd=WWpCeEJYektDcTc2Zm1tK29RSXFUZz09
Meeting ID: 976 9645 4919
Passcode: 238560