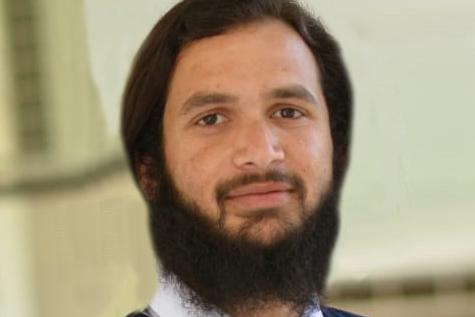
Event date:
Mar
8
2021
9:00 am
Autonomous Navigation of an Unmanned Aerial Vehicles (UAV) in a Simulated Canal Environment using Deep Reinforcement Learning
Supervisor
Dr. Abubakr Muhammad
Dr. Talha Manzoor
Student
Tallat Mahmood
Venue
Zoom Meetings (Online)
Event
MS Thesis defense
Abstract
The agriculture sector contributes a major impact on the GDP of Pakistan and plays a vital role in the economy of the country. The productivity of the agriculture sector highly depends on an efficient irrigation system consisting of canals (outflows of dams, rivers, and barrages). An efficient irrigation system requires proper inspection and maintenance. Usually, the inspection of the canals is performed manually which is an infeasible, time-consuming, and laborious task that affects the growth of the agriculture sector. To deal with these issues, a possible solution is to perform autonomous inspection and monitoring of the canals. An autonomous inspection is performed by navigating the Unmanned Aerial Vehicles (UAVs) equipped with different sensors like LiDAR and stereo cameras.
The existing autonomous navigation systems require a 3D map of the environment to find an obstacle-free path for UAVs. The 3D map and obstacle-free path planner algorithms require on-board high computational resources and memory. The UAVs lack these resources due to the small size, complex dynamics constraints, and limited power resources. To deal with these issues, we propose a deep reinforcement learning-based approach for autonomous canal navigation. In the proposed approach, we learn the action policy using observation (depth image) and reward (feedback) from the environment to navigate the UAV robustly without the 3D map of the canal. We have simulated a real canal-like environment in the Unreal Engine framework. We learn action policies using Deep Q Network (DQN) and double Deep Q Network (double DQN) algorithms to reach a waypoint (GPS coordinate) of the canal. The test analysis of the learned policies for navigation shows that the double DQN outperforms for seen and unseen parts of the canal. So, the double DQN is used to train the UAV over the complete canal. In this setup, the complete canal is divided into equidistant waypoints. The canal navigation is achieved by reaching these waypoints sequentially. The immediate next waypoint is considered as the goal point. The comprehensive analysis of this setup concludes that patch-wise training is required to navigate the complete canal. The experimental results of the proposed scheme show that the UAV can navigate the complete canal keeping a safe distance from obstacles without having a 3D map of the canal.
The existing autonomous navigation systems require a 3D map of the environment to find an obstacle-free path for UAVs. The 3D map and obstacle-free path planner algorithms require on-board high computational resources and memory. The UAVs lack these resources due to the small size, complex dynamics constraints, and limited power resources. To deal with these issues, we propose a deep reinforcement learning-based approach for autonomous canal navigation. In the proposed approach, we learn the action policy using observation (depth image) and reward (feedback) from the environment to navigate the UAV robustly without the 3D map of the canal. We have simulated a real canal-like environment in the Unreal Engine framework. We learn action policies using Deep Q Network (DQN) and double Deep Q Network (double DQN) algorithms to reach a waypoint (GPS coordinate) of the canal. The test analysis of the learned policies for navigation shows that the double DQN outperforms for seen and unseen parts of the canal. So, the double DQN is used to train the UAV over the complete canal. In this setup, the complete canal is divided into equidistant waypoints. The canal navigation is achieved by reaching these waypoints sequentially. The immediate next waypoint is considered as the goal point. The comprehensive analysis of this setup concludes that patch-wise training is required to navigate the complete canal. The experimental results of the proposed scheme show that the UAV can navigate the complete canal keeping a safe distance from obstacles without having a 3D map of the canal.
Zoom Link: https://zoom.us/j/95834674846?pwd=N01CbEJzR3RpdUtrcWtOVkV5eGJjQT09
Meeting ID: 958 3467 4846
Passcode: 629508