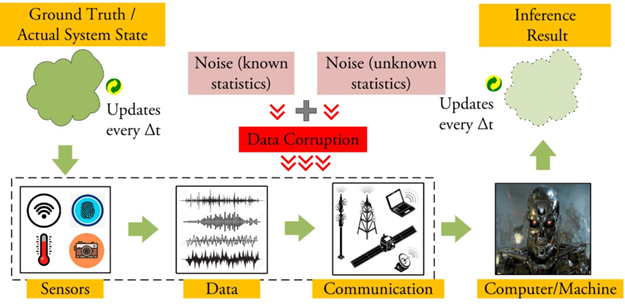
Event date:
Mar
12
2021
5:00 pm
Robust Filtering – Novel Statistical Learning and Inference Algorithms with Applications
Supervisor
Dr. Muhammad Tahir
Dr. Momin Uppal
Student
Aamir Hussain Chughtai
Venue
Zoom Meetings (Online)
Event
PhD Synopsis defense
Abstract
The last few decades have witnessed the arrival of technologies that only seemed a fantasy a century ago. We have seen tremendous progress in mobile communication, personal computing, manufacturing, and automation, sensing apparatus, etc. With the advent of enabling technologies, the world is now bracing for the fourth industrial revolution. It is based on a fusion of different technologies blurring the boundaries between the physical, digital, and biological spheres. The key elements in this revolution are the proliferation of data, methods to exploit it efficiently, and the availability of increased computation power. Having been able to mimic the ability of inference, the hallmark of intelligent species, we have seen remarkable popularization of Artificial Intelligence, Machine/Deep Learning, Neural Information Processing, Data Analytics, etc., and significant advances in statistical methods and signal processing algorithms. A key question for using data effectively is to estimate/determine the model, structure, or latent state that describes the data. This is what we refer to as the learning, inference, or estimation problem. With real-time requirements, the problem can be challenging and remains an active research area in different fields. The problem can further be aggravated when the data is corrupted with different abnormalities having unknown statistics. This work aims to investigate and develop robust learning and estimation theory and methods to mitigate the effect of measurement contaminations. In particular, we intend to study the nonlinear filtering problem, i.e. a real-time inference problem, in the presence of observation contaminations. Studying the effect of data corruption on state estimation, we plan to develop novel methods for estimation error reduction for real-world applications.
Zoom Link: https://zoom.us/j/95801701715?pwd=YkZoK2RHa1BXM29iVlFRb2lXVU96dz09
Meeting ID: 958 0170 1715
Passcode: 957018
Publications:
- A. H. Chughtai, M. Tahir and M. Uppal, "A Robust Bayesian Approach for Online Filtering in the Presence of Contaminated Observations," in IEEE Transactions on Instrumentation and Measurement, vol. 70, pp. 1-15, 2021, Art no. 9503215, doi: 10.1109/TIM.2020.3033759.
- A. H. Chughtai, U. Akram, M. Tahir and M. Uppal, "Dynamic State Estimation in the Presence of Sensor Outliers Using MAP-Based EKF," in IEEE Sensors Letters, vol. 4, no. 4, pp. 1-4, April 2020, Art no. 7001204, doi: 10.1109/LSENS.2020.2983453.
- A. H. Chughtai, M. Tahir and M. Uppal, "Outlier-Robust Filtering For Nonlinear Systems With Selective Observations Rejection," Under Review, IEEE Signal Processing Letters.