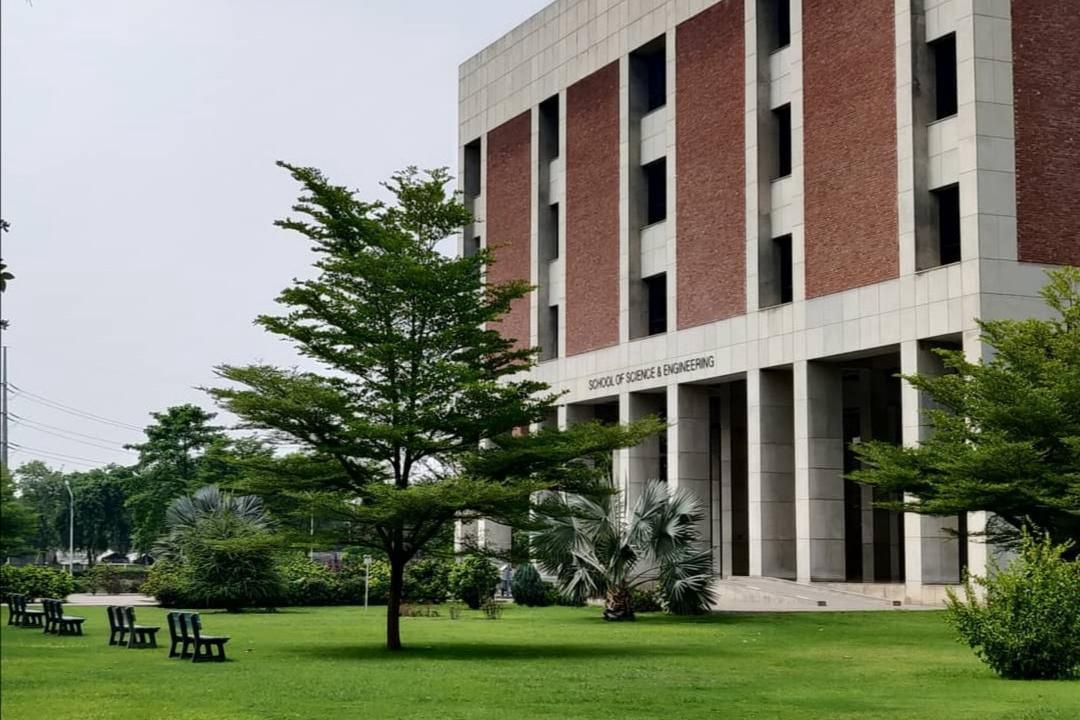
Event date:
Feb
26
2021
11:00 am
RKF-ELM: A Robust Kalman Filter based Extreme Learning Machines for Accurate Heart Rate Estimation
Supervisor
Muhammad Tahir
Student
Muhammad Nabeel Tahir
Venue
Zoom Meetings (Online)
Event
MS Thesis defense
Abstract
Wearable computing has introduced innovative approaches and products in entertainment, sports, industrial logistics, and many other areas. However, no other field is anticipating and integrating wearable technology so broadly as healthcare, with interests ranging from well-being and disease prevention to chronic patient care and cross-cutting all medical disciplines. A typical healthcare wearable can collect data for multiple physiological signals, for example, an electromyogram (EMG), motion signals, electrocardiogram (ECG) signals, electroencephalogram (EEG) signals, and photoplethysmogram (PPG) signals. We aim to utilize only PPG signal for further processing and estimation of the human heart rate which is one of the most readily accessible and informative vital signs to evaluate cardiovascular conditions. Since PPG sensors are not only used in healthcare devices but also in the fitness trackers as a guide to monitor heartbeat rhythms. It is important to accurately measure and estimate the heart rate from PPG signals as it might affect the performance of an athlete or the medical history of a patient. The PPG captures the change in blood volume during cardiac cycles and the light intensity in tissues and other non-pulsatile blood. Each cardiac cycle appears as a peak in the signal, which reflects heart activities. However, the motion artifact resulting from the movements of subjects mostly contaminates the PPG signals. This, in turn, poses great challenges on an accurate heart rate estimation. Motion artifacts can be modeled as unknown signals corrupting the useful PPG signal. We resort to extreme learning machines (ELMs) for real-time learning of complex features and benefit from the robustness and high accuracy of ELMs. The proposed algorithm (RKF-ELM) exploits the robust Kalman filter to remove the outliers and smooth the final HR estimate. The performance of the (RKF-ELM) has been thoroughly compared with the existing state-of-the-art algorithms on a publicly available IEEE dataset of 23 subjects. On PPG recording of all the subjects, the algorithm achieves the results in an average absolute error of 0.98 BPM. The error of the (RKF-ELM) algorithm has significantly reduced from the previously existing techniques. The system has proved to be accurate in the presence of intense motion artifacts and requires fewer trainable parameters as compared to alternatives. The algorithm requires low computational resources and can be implemented in wearable health and fitness trackers without any modifications.
Join Zoom Meeting: https://zoom.us/j/92532083644?pwd=YkRlREJLb202dC96aWpMU1JuaWpWZz09
Meeting ID: 925 3208 3644
Passcode: 250109