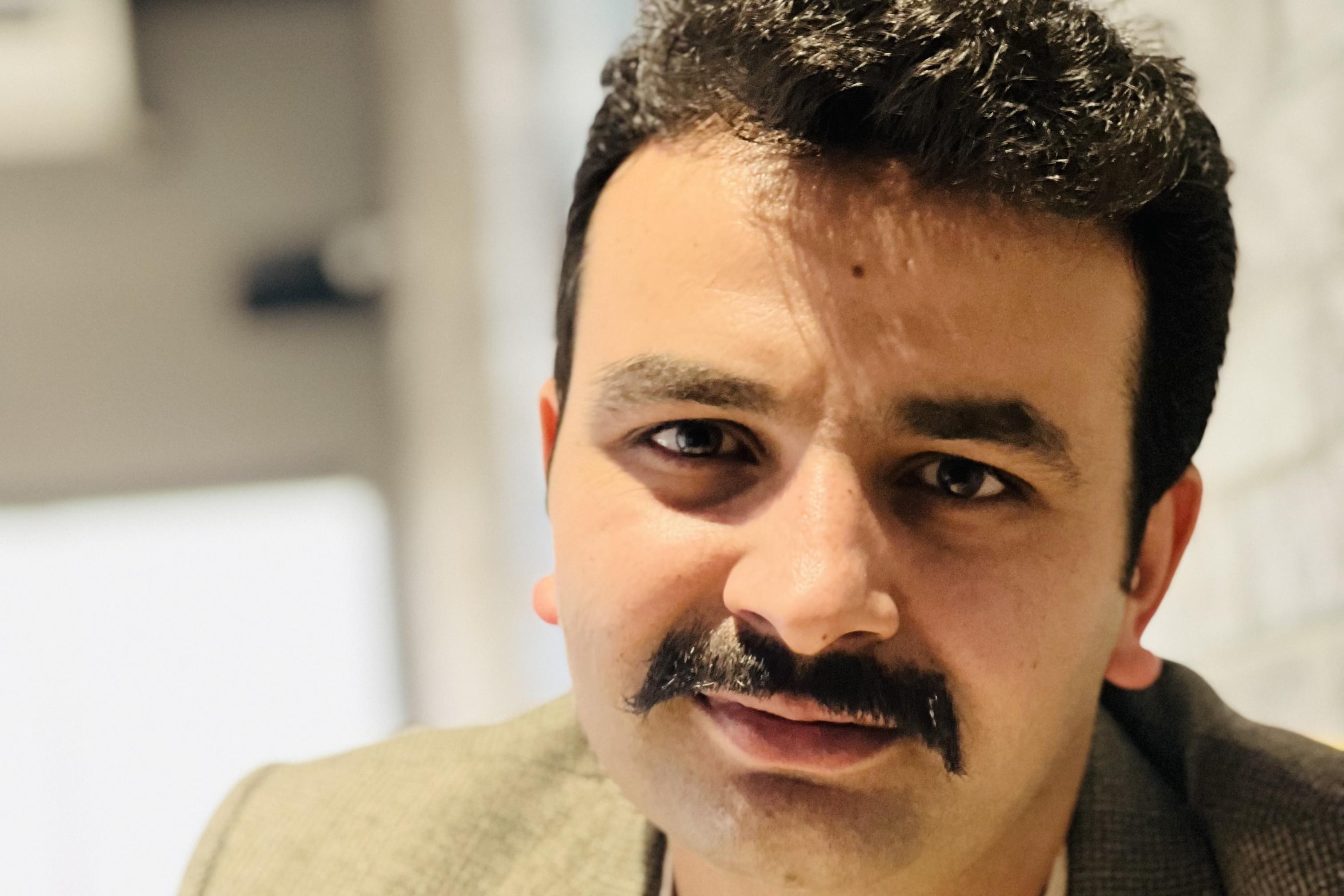
Towards a Shallow Sparse Autoencoder Framework for Epileptic EEG Analysis
Abstract:
This thesis intends to explore high performance but computationally efficient frameworks for bio-potential signal processing especially in connection to Epileptic EEG signal analysis. EEG signal analysis has been of interest for the purpose of diagnosis of Epilepsy at the hospital and monitoring the effectiveness of treatment in the follow-up visits. It is also of interest in case of ambulatory patients for extended diagnosis and/or patient-care. Diagnosis entails detection of seizure events and identification of epilepsy types in the recorded EEG from the patient. Patient-care encompasses need to predict and detect seizures at the patient-end in the EEG captured by the sensor. This can be used for alerting the patient in advance and for transmission of warnings and the actual EEG signal to the care-provider.
Requirements from a Neurologists support system in dealing with epileptic patients’ EEG records include accuracy in localization of epileptic events, customization of the system according to neurologists’ style of diagnosis, summarization of important events, less storage space, good fidelity of stored signal for later use, computational efficiency and finally a user-friendly system interface. To enable the widespread use of wearble devices by ambulatory patients, the targeted features are smaller form-factor, low energy consumption, improved accuracy, trainability, patient personalization and smartness in meeting the patient’s and doctor requirements. Storage and/or transmission of EEG signal in the compressed form is a basic requirement in any of these applications.
This research explores the merit of a signal processing framework with processing steps shared among the desired EEG analysis tasks. This can reduce overall computational effort and also enable closer cooperation such as for data selection and adaptive compression. In this regard traditional and new techniques and their hybrids are being explored. One direction of work has resulted in an extended i-NSS system as a Neurologists support system based on DWT-transformed data used both for classification and compression based on traditional techniques. This synergy not only results in data reduction and adaptive compression as per importance of data but also allows maintaining the classification fidelity of reconstructed signal greater than 99% with the classification results obtained on the original data. In one of our work we have further extended i-NSS to distinguish between four types of epilepsies.
We have also experimented with a trainable hybrid approach involving shallow autoencoder (AE) and a conventional classifier for single channel EEG signal. This has led to SVM based classification results for seizure detection with accuracy, sensitivity and specificity as high as 99.2%, 96.1% and 99.2% respectively. This exceeds the best results reported and with lowest computational complexity. This framework is interesting for the development of smart EEG wearable device for the patient. Experiments on compression, seizure prediction and epilepsy type classification under the same framework are ongoing.
PhD Committee:
- 1.Dr Nadeem Ahmad Khan(Supervisor)
- 2.Dr Muhammad Awais Bin Altaf
- 3.Dr Hassan Mohy Ud Din
Publications:
- G. H. Khan, N. Ahmad Khan, M. A. Bin Altaf and M. Ur Rehman Abid, "Classifying Single Channel Epileptic EEG data based on Sparse Representation using Shallow Autoencoder", 43rd Annual International Conference of the IEEE Engineering in Medicine & Biology Society (EMBC), 2021, pp. 643-646, doi: 10.1109/EMBC46164.2021.9630714. (Conf. Paper)
- G.H. Khan, Hashmi, M.A., M.M. Awais, N.A. Khan an, R.B. Ahmad, “High Performance Multi-class Motor Imagery EEG Classification” BIOSIGNALS 2020, pp. 149-155. (Conf. Paper)
- S. Shakeel, N. Afzal, G. H. Khan, N. A. Khan, M. u. R. Abid and M. A. B. Altaf, "EDM: A multiclassification support system to identify seizure type using K Nearest Neighbor", 28th IEEE International Conference on Electronics, Circuits, and Systems (ICECS), 2021, pp. 1-4, doi: 10.1109/ICECS53924.2021.9665565. (Conf. Paper)
- N. A. Khan, G. H. Khan, M. A. Ahmad, M. A. bin Altaf and M. O. Tarar, "The Extended i-NSS: An Intelligent EEG Tool for Diagnosing and Managing Epilepsy", In International Joint Conference on Biomedical Engineering Systems and Technologies, pp. 243-262. Springer, Cham, 2020. (Book Chapter)