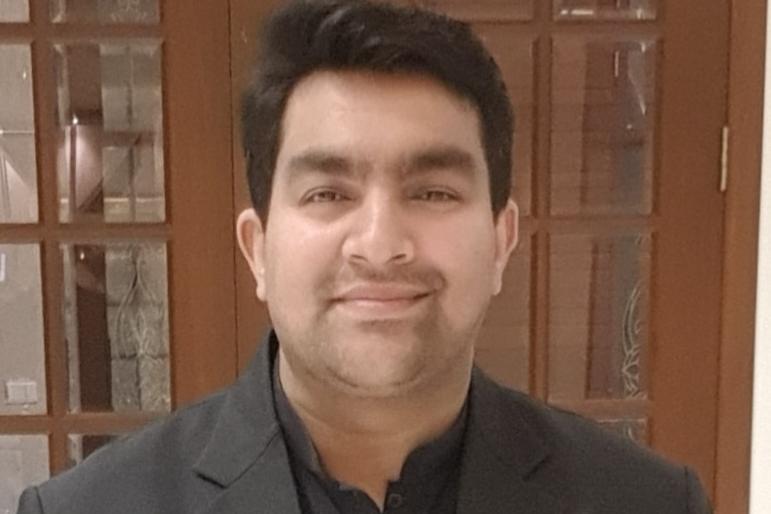
Frequency Division Multiplexed Analog Front End for Electroencephalogram Recording Systems
Abstract:
Over the last decade, there is tremendous progress in understanding the working of the brain activities using Electroencephalogram (EEG) signals. EEG signals are being used in detecting disorders such as epilepsy, narcolepsy, trauma and these signals are also being used in the control of prosthetic devices. These systems are multi-channel, bulky, power expensive, and require a medical setting for the monitoring of patient data. There is a need for a wearable EEG acquisition system that could record multiple channels keeping the power and noise requirements of this system low. Analog front end (AFE) contains instrumentational amplifier (IA), and programmable gain (PG) stage, the IA is extremely critical as it is the most power-hungry block to keep the noise requirement low for the acquisition of EEG signals.
The goal of this work is to develop an energy-efficient AFE to acquire clean EEG signals. Frequency division multiplexing will be utilized to share the most power-hungry blocks of the AFE while keeping intact the signal-to-noise ratio. The front end will be simulated, designed, and fabricated using a 180nm CMOS process. The proposed system will ensure a small form factor and low noise while multiplexing at least 2-channels per AFE, and solve the 1) 50/60 Hz common-mode interference, 2) in-band noise (mainly 1/f noise and electrode DC offset), and 3) limited input impedance of the IA, in a wearable environment. The target is to achieve power, and an input-referred noise of less than 150µW/channel and 5 µVrms [0.5-100 Hz], respectively, while operating at 1.8V.Evaluation Committee:
- Dr. Muhammad Awais Bin Altaf (Supervisor)
- Dr. Nauman Zaffar Butt (Evaluator)
Zoom Link: https://lums-edu-pk.zoom.us/j/95567738719?pwd=NzhJMjRkSDRSOTdZazhwYzFqQXh6UT09
Meeting ID: 955 6773 8719
Passcode: 337710