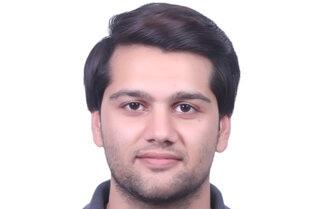
Analysis of Remote Sensing with GATs
Abstract:
According to UNDP, population growth in the cities sprung out from 54.6% to 78.3% between 1950 and 2015. Population growth is the main driver for the increasing rate of urbanization and deforestation. Urbanization induces Land-Use/Land-Cover transformations of replacing soil, vegetation, forests, and directly impart land surface temperature (LST). Deforestation poses risks to the climate, biodiversity, and food security by degrading the ecosystem. Efficient monitoring of land transformations is needed to effectively address the issues of climate change and global warming associated with urbanization and deforestation.
With the advent of frequent satellite imagery, it is now possible to monitor the aforementioned changes remotely. Deep learning on graphs has opened new research avenues to generalize classical deep learning concepts to irregularly structured data. Our proposed method is a fast and cost-efficient way of doing analysis of remote sensing data, which in turn allows the institutions to monitor, take timely decisions and policymaking. We employ a novel method to classify Spatio-temporal land transformations from satellite images. Region Adjencancy Graphs (RAGs) are made by using superpixels as nodes. We combine those RAGs in what we call “Temporal RAG” from subsequent years to incorporate temporal relations in graphs. We use Graph Attention Networks (GATs) to learn the Spatio-temporal relation between temporal RAG superpixels. Finally, we predict one of four Land Use/Land Cover transformations namely construction, destruction, cultivation, de-cultivation. We used the Asia14 dataset, consisting of 4578 satellite images of ~1526 locations from 3 years (2011, 2013, 2017). We ran MoNet (GCN) to produce baseline results on the MNIST dataset and successfully replicated the 97.3% accuracy, which is proof of concept that images can be classified using GNNs.