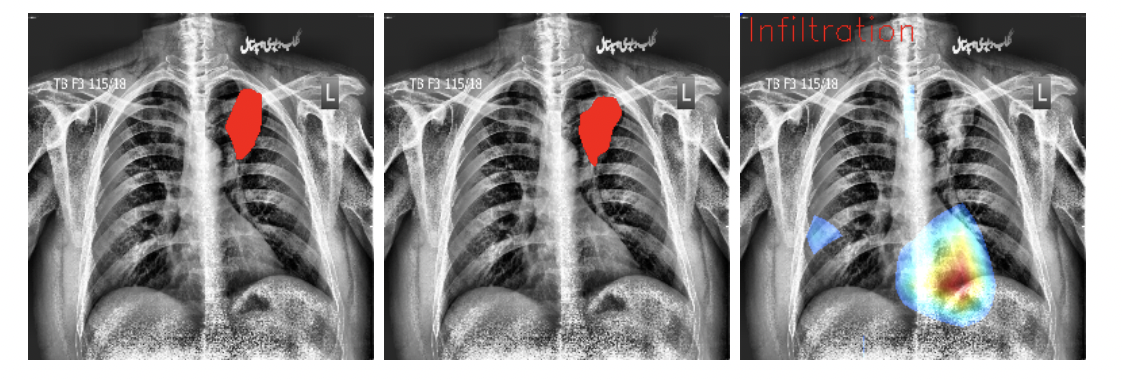
Mimicking Radiologists: Localization Of Diseases In Chest X-Rays Using Deep Learning Methods
Abstract:
Lung diseases have remained at the tip of health problems for over decades. Whether it's common flue infections like the Influenza virus or severe conditions like Pneumonia or COVID-19, chest x-rays play a crucial role in diagnosing and treating all such diseases. X-rays are long known most inexpensive and convenient method of getting a picture of lung tissues and analyzing the extent to which they are affected or locating any abnormality in them. However, interpreting such x-rays require highly qualified professional and capable radiologists who might not be available easily in many areas. Moreover, accessing this massive number of patients’ data is undoubtedly a tedious task for doctors, and providing any automated assistance would be appreciated by them as well.
In this regard, most work done includes deep learning for detecting different diseases from chest x-rays, but there is little work for localization in chest x-rays. A typical radiologist approach is to see patches referred to as regions of interest (ROI) where abnormality occurs in a chest x-ray. These findings diagnose whether a person is suffering from Pneumonia, COVID-19, or any other lung disease.
This thesis work revolves around the localization of diseases in chest x-rays. The dataset used is novel and collected recently from Gulab Devi Hospital comprising of hundreds of patient's data collected at different time stamps having disease annotations from specialist doctors. We propose localization via local detectors and localization via semantic segmentation. To further improve results, we performed data augmentation and pre-processing. We have also leveraged transfer learning and achieved an IoU of 0.926. The F1-score is also improved from 0.00044 to 4.065, which is significantly higher and comparable with the state-of-the-art CheXNet classification results.
Evaluation Committee
- Dr. Murtaza Taj (Supervisor)
- Dr. Muhammad Fareed Zaffar (Evaluator)