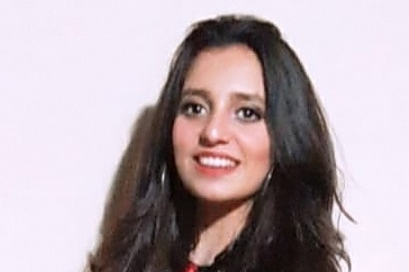
Comparative Analysis of Stochastic Learning Dynamics: An Evolutionary Approach
Abstract:
Stochastic Best Response (SBS) and Stochastic Better Response (SBT) are two popular and widely recognized decision-making strategies of rational agents in population games. The two decision strategies differ considerably in their resource requirements in order to make decisions and have distinct update rules, but their steady state behavior is identical for most games that capture important practical application fields. The traditional literature on learning dynamics is generally focused on analysis of the steady state behavior of population under different strategies. Given the fact that SBS and SBT have similar steady state behavior, comparison of these dynamics using traditional tools does not provide conclusive results. In this thesis we conduct detailed comparative analysis of SBS and SBT in a competitive evolutionary framework for the class of 2x2 symmetric games. We present theoretical analysis of the effect of the payoff structure of the game on the stochastic stability of these strategies. We establish that for class of two action symmetric game, SBT dominates SBS if the payoffs of a player associated with each pair of corresponding joint action profiles have opposite relationships. No strategy dominates when these payoffs are equal. For the remaining exhaustive relationships between corresponding payoffs, SBS dominates SBT. We also examine the stochastically stable states for all payoff-based categories of symmetric games when players have synchronous update rule. In the field of game theoretic learning, this analysis of stochastically stable joint action profiles is of individual interest.
Evaluation Committee:
Supervisor: Dr. Hassan Jaleel
Evaluator: Dr. Abubakr Muhammad
Zoom Link: https://lums-edu-pk.zoom.us/j/92176973038?pwd=VWNpenRkNjVvdW4rcHZLZVp3SzRydz09
Meeting ID: 921 7697 3038
Passcode: 506045