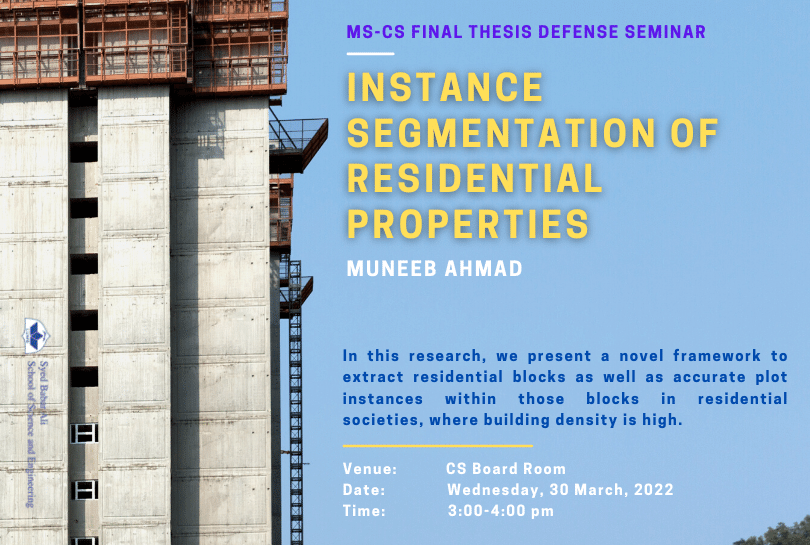
Instance Segmentation of Residential Properties
The performance of object instance segmentation in remote sensing images has been greatly improved through the introduction of many landmark frameworks based on convolutional neural network. However, segmentation of closely built properties with variable sizes and no definite pattern, a common trait in developing countries, is still a challenging task. Accurate segmentation information of residential and commercial properties built or non-built in a given region is of high value for many applications e.g. estimation of disaster damage and restoration, calculation of property tax, analysis of urban growth and population density in less-developed countries. In this research, we present a novel framework to extract residential blocks as well as accurate plot instances within those blocks in residential societies, where building density is high. We employ the state-of-the-art instance segmentation algorithm, Mask R-CNN as a backbone framework and further improve the segmentation results by adding a grid estimation approach that utilizes different aspects of statistical information from the Mask-RCNN predictions to overcome the issues of high variability in structure and building patterns. We also provide a large annotated dataset containing over 800 images of densely built societies in different cities in Pakistan.
Evaluation Committee:
Dr. Murtaza Taj (Supervisor)
Dr. Fareed Zafar (Evaluator)