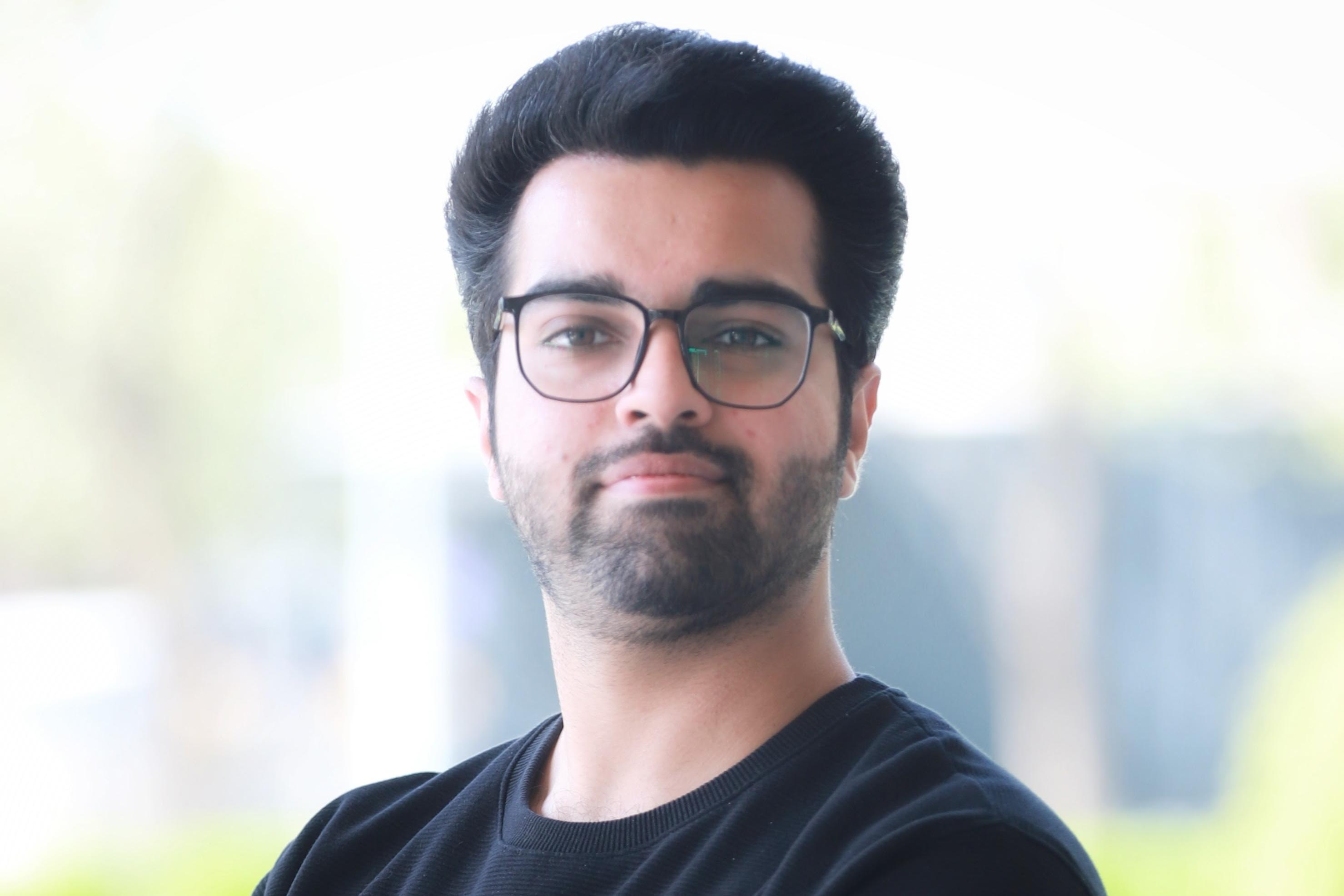
Paying attention to the radiologists
Abstract:
According to the Association of American Medical Colleges, by 2034, America’s shortage of radiologists and other medical specialists could exceed 35,000. The situation is equally tight in the UK, with just 2% of radiology departments able to fulfill their imaging reporting requirements within contracted hours in 2018. Keeping in mind this lack of resources in medical imaging and the fact that Pakistan has the 10th highest annual death rate for patients dying from lung diseases, there is a dire need for successful automation in medical imaging to ameliorate this situation. If not totally automated, Computer-aided diagnosis (CAD) can be at least interpreted as a second opinion in assisting the physicians during diagnosis. For diagnosis, Chest X-Rays (CXRs) are the cheapest and most commonly utilized tool used by radiologists for lung diseases. So, in this thesis, we are trying to understand how Radiologists diagnose different abnormalities using disease patches on CXRs. Much work is being done on automated diagnosis, but the acceptability of these methods into routine clinical practice requires an explanation from the models as to why one should trust them. In order to build trust between patients and providers, transparency, interpretability, and explanation are essential.
This thesis involves the classification and localization of numerous lung diseases in CXRs. The dataset being used is from a local hospital comprising around 500 images and disease annotation data of 233 patients collected at different timestamps. We propose using a pre-trained deep convolutional neural network as a base model and attaching an attention-based mechanism to increase the model’s performance and explainability. After the classification results, we employed a saliency mapping technique called GradCAM to extract the localization results from the model. Our proposed model trained on the Gulab Devi dataset achieves an AUROC classification score of 0.7453 on five different lung diseases, improving 10.04% over the results of the state-of-the-art model on the Gulab Devi dataset. Qualitative results for the localization also show an improvement using our proposed model.
Evaluation Committee:
Dr. Murtaza Taj (advisor)
Dr. Muhammad Fareed Zaffar