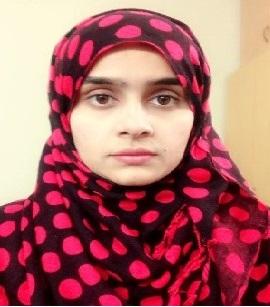
DeepRISLoc: WiFi Indoor Positioning System Harnessing Reconfigurable Intelligent Surfaces and Deep Learning for Enhanced Environment Adaptability
Abstract:
Indoor localization has emerged as an essential research area due to the limitations of Global Navigation Satellite Systems (GNSS) in indoor environments and the growing demand for accurate positioning in various applications, such as navigation, emergency response, and context-aware services. WiFi fingerprinting is a promising technique for indoor localization, leveraging the widespread deployment of WiFi infrastructure. However, its performance is often affected by the complex indoor radio propagation environment and the time-varying nature of wireless channels. This research investigates the use of Reconfigurable Intelligent Surfaces (RIS) and Deep Learning (DL) techniques to enhance the performance of WiFi fingerprinting-based indoor localization systems.
The research is divided into two parts, focusing on Indoor Positioning Systems (IPS) without RIS and with RIS integration. In both parts, we apply DL techniques and compare their performance with traditional positioning models. The first part of the research explores the application of DL methods, specifically Convolutional Neural Networks (CNN), in fingerprint-based localization without RIS. A comparative study is conducted to evaluate the performance of these DL models against traditional positioning algorithms in various scenarios.
The second part of the research introduces the concept of RIS, a novel class of intelligent surfaces capable of controlling electromagnetic waves. We propose a framework integrating RIS into existing WiFi infrastructure to improve localization accuracy by adaptively shaping the wireless channel. At first, we optimized the RIS functionality in an indoor environment.
We revisited the existing analytical propagation model and proposed a Hybrid End-to-End (E2E) model. The proposed hybrid model combines the benefits of the analytical model and Full wave simulation (CST), providing a quick and accurate estimation of RIS response without sacrificing accuracy.
In our future research work, we plan to develop an experimental framework for a RIS-enabled IPS. The primary objective of this work is to construct an extensive dataset that will enable us to systematically evaluate the performance of the proposed system. We intend to employ advanced DL models to train and test both the RIS-enabled and without RIS IPS systems. Through this comparative analysis, we hope to demonstrate the potential advantages of incorporating RIS technology, ultimately resulting in significantly enhanced positioning accuracy for indoor environments.
Publications:
- Javed, Aisha, Naveed Ul Hassan, and Chau Yuen. "Accurate and Stable Wi-Fi based Indoor Localization and Classification Using Convolutional Neural Network." 2021 IEEE VTS 17th Asia Pacific Wireless Communications Symposium (APWCS). IEEE, 2021. (Conf. Paper)
- Javed, Aisha, and Naveed Ul Hassan. "Low-Effort Deep Learning Method Trained through Virtual Trajectories for Indoor Tracking." 2021 IEEE 32nd Annual International Symposium on Personal, Indoor and Mobile Radio Communications (PIMRC). IEEE, 2021. (Conf. Paper)
- Javed, Aisha, et al. "Revisiting End-to-End Communication Model for Realistic Reconfigurable Intelligent Surfaces." 2022 IEEE International Symposium on Antennas and Propagation and USNC-URSI Radio Science Meeting (AP-S/URSI). IEEE, 2022. (Conf. Paper)
- Aisha Javed, Ammar Rafique, Naveed-Ul-Hassan, Muhammad Zubair, “A Hybrid Approach for End-to-End Propagation Modeling in Reconfigurable Intelligent Surfaces Assisted Communications”. (Journal Paper) (to be submitted)
PhD Committee:
1.Dr. Naveed-Ul-Hassan (Supervisor)
2.Dr. Hassan-Mohy-Ud-Din
3.Dr. Ijaz Haider Naqvi