Winners of the second cohort 2024
The winners of the second cohort of the Khalid and Mussarrat Aziz Research Grants for Planet Earth are:
Brief summaries of the funded proposals are given below:
Integrating population genetics and metagenomics for advancing regenerative wheat farming
Dr Zaigham Shahzad, Dr Khurram Bashir, and Dr Muhammad Tariq
Food security and climate change are critical challenges facing our planet. Modern agricultural practices have increased crop yields but rely heavily on synthetic fertilizers, tillage, and energy consumption, leading to greenhouse gas emissions and soil degradation. These issues threaten long-term food security and ecosystem health. Regenerative agricultural practices, which emphasize reducing agricultural inputs and minimizing soil disturbance, offer promising solutions to enhance soil health and carbon sequestration. Given the global importance of wheat, the optimal cultivation of this crop is key to transitioning to regenerative farming. However, most modern wheat cultivars perform poorly under regenerative settings. To address this, our team is employing quantitative genetics approaches using a historical wheat population to discover genetic factors influencing wheat adaptability of regenerative farming. These genetic mechanisms can be leveraged to expedite the development of wheat varieties suited for cultivation under regenerative agricultural settings. Additionally, we aim to promote sustainable agriculture through small farms and identify the microbes that contribute to soil health, plant growth, and human health. This project will uncover the genetic and microbial factors that govern regenerative wheat farming, providing comprehensive insights into the biological mechanisms that can contribute to a more sustainable and resilient agricultural future.
Flood Risk modelling: Using generative adversarial networks (GAN) multi-risk flood impact visualization
Dr Muhammad Tahir
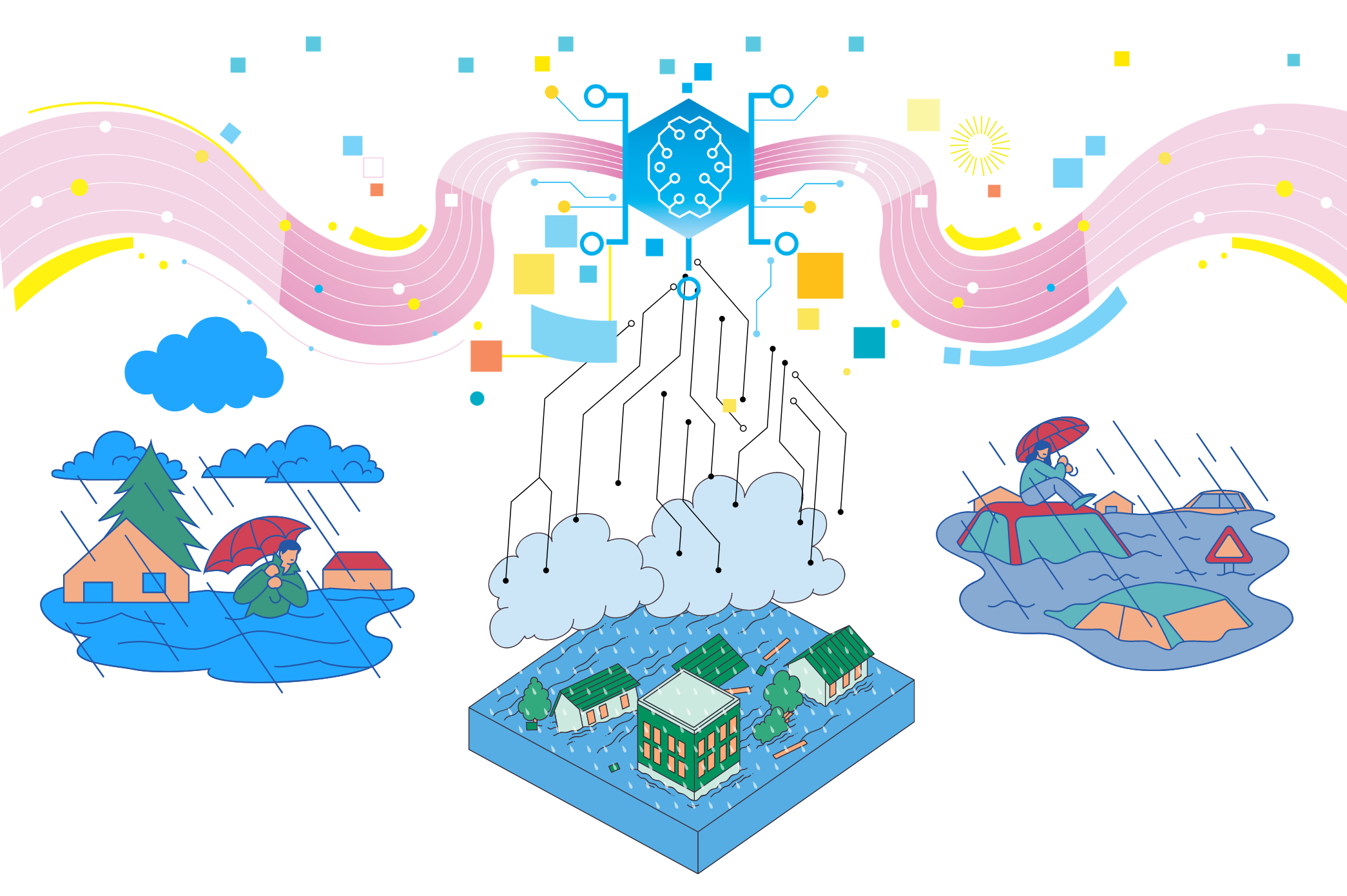
Flooding in Pakistan, largely attributed to intense monsoon rainfall, is becoming a recurring natural calamity indicative of climate change, causing irremediable loss to infrastructure and human lives. Fast and accurate flood impact assessment is a crucial factor in disaster prevention management and operational strategies, especially when human lives are at stake. Recently, flood prediction science has begun to use machine learning methods to emulate the physics-based simulations of hydrodynamics by learning the target systems regardless of their physical relationships. Building on the advances in generative capabilities of large vision models, this work seeks to investigate the use of a predictive vision model to generate post-flood images from existing pre-flood images using earth data, satellite imagery, and surveillance data, integrated with ML/AI tools to infer flood extent and severity from a spatiotemporal lens. More specifically, we seek to answer the question: can AI models be used to generate accurate predictive visualizations of extreme events like floods, to inform disaster prevention and management? This type of predictive visualization of flooding as a climate impact can be integral for policy formulation to raise environmental awareness and facilitate dialogue on long-term climate adaptation decisions.