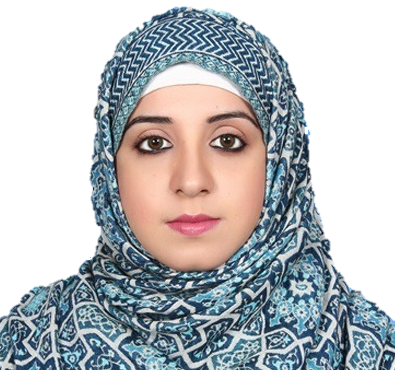
A Noninvasive Glucose Monitoring System Using a Single-Wavelength LED
Abstract:
Diabetes and impaired glucose tolerance are some of the most prevalent diseases in the world. Approximately 425 million people around the world are diabetic and 1 in 6th births is affected by hyperglycemia in pregnancy. Continuous blood glucose level (BGL) monitoring is essential for diabetic patients and pregnant women to avoid diabetes-related complications. Conventional glucose monitoring methods around the world are invasive, painful, costly, and time-consuming. The past few decades have seen developments made in designing semi or minimally invasive to noninvasive glucose monitoring systems. Semi-invasive systems mostly are based on electromechanical technology for glucose measurements and the devices need to be implanted by medical professionals. Minimal invasive devices include electrochemical patches placed on the skin for glucose measurements. Additional features like continuous glucose monitoring and smartphone displays have also been successfully designed. However, these devices are expensive, a bit complex and uncomfortable to use and have low accuracy compared to the conventional invasive technique of glucose measurement. This PhD thesis has aimed to develop a cost-effective, pain-free, and easy-to-use noninvasive glucose monitoring SoC, that can frequently measure blood glucose levels. The proposed system SoC has three main modules. The LED driver, PPG readout circuit and glucose prediction processor. The glucose prediction processor eliminates noise and baseline drifts from recorded PPG signals and extracts fourteen distinct features. These features are utilized in training models using Support Vector Regression (SVR). The trained models are then used to predict blood glucose levels. The best-performing training model has been selected for hardware implementation. The designed SoC is then verified on 200 subjects. The dataset has been collected in two phases. For the dataset collected in the first phase of the research work, the SoC is designed using Fine Gaussian Support Vector Regression (FGSVR) as a trained model. Six distinct features extracted from the PPG signals are utilized for glucose estimation. The obtained mARD (mean absolute relative difference) for the test data is 7.62% with an RMSE (root mean square error) of 11.20. The system consumes a total power of 1.62 mW. For the dataset collected in the second phase of research, the number of subjects has increased with more diabetic patients. An improved SoC is successfully realized. It consumes less power, which is 186 μW for the overall system. Non-medium Gaussian Support Vector Regression (NMGSVR) trained model using ten distinct features from PPG signal are utilized for glucose estimation. The obtained mARD (mean absolute relative difference) for the test data is 6.9% with an RMSE (root mean square error) of 10.20.
List of Publications:
Journals:
1. Aminah Hina and Wala Saadeh, “A Noninvasive Glucose Monitoring SoC Based on Single Wavelength Photoplethysmography,” IEEE Transactions on Biomedical Circuits and Systems (TBioCAS), vol 14, no. 3, pp 504-515, July 2020.
2. Aminah Hina and Wala Saadeh, “Noninvasive Blood Glucose Monitoring Systems Using Near-Infrared Technology—A Review,” Sensors, vol 22, no. 13, 4855, June 2022.
3. Aminah Hina and Wala Saadeh, "A 186μW Photoplethysmography-Based Noninvasive Glucose Sensing SoC," in IEEE Sensors Journal, vol. 22, no. 14, pp. 14185-14195, July 2022.